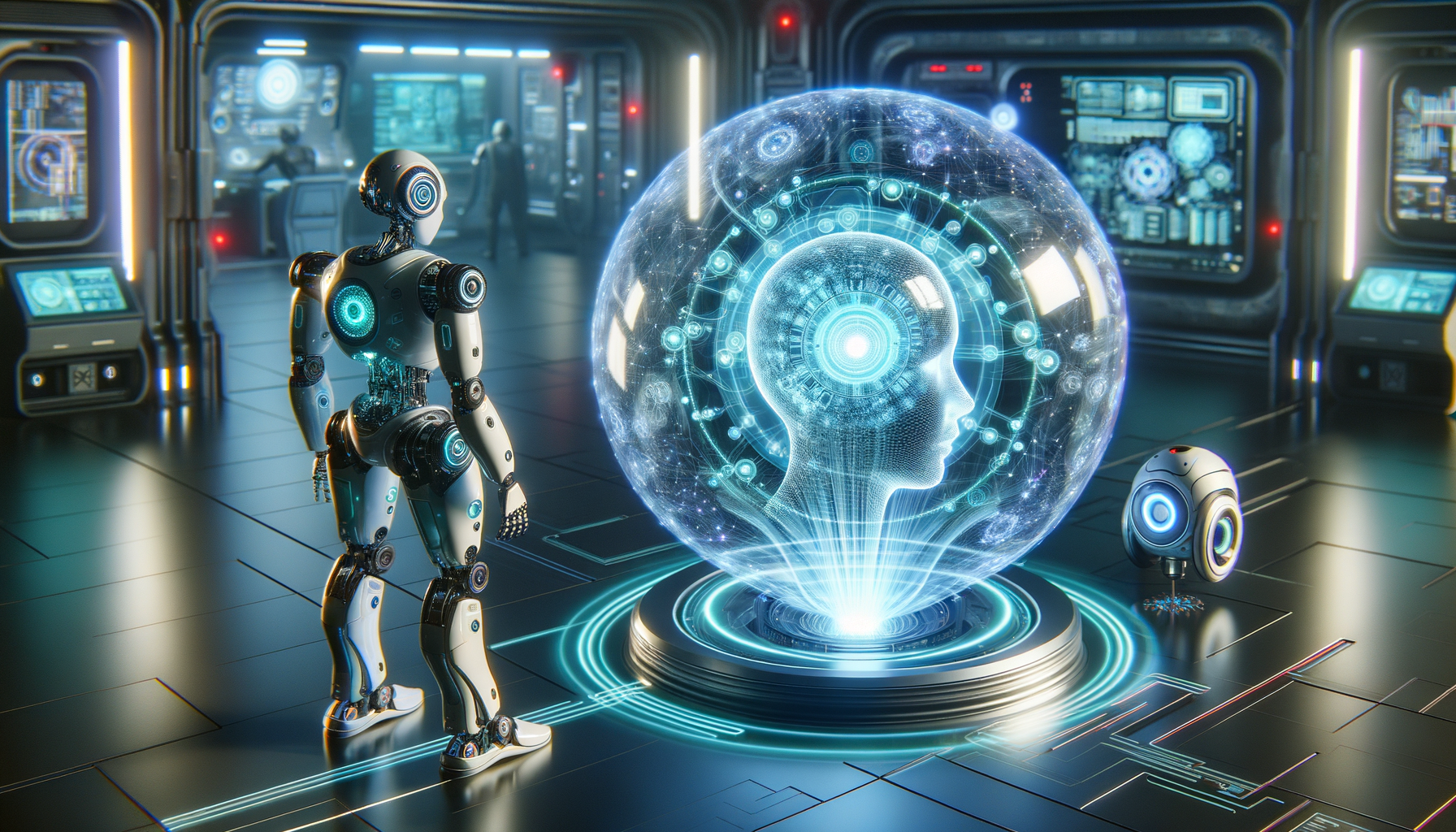
Understanding and Protecting Against Adversarial Attacks on Large Language Models like OpenAI’s GPT-4
In the rapidly evolving landscape of artificial intelligence, large language models such as OpenAI’s GPT-4 have made significant strides in understanding and generating human-like text. However, as these models become more integrated into various aspects of our digital lives, they also become targets for adversarial attacks. These attacks are designed to exploit weaknesses in the models, potentially leading to misinformation, biased outputs, or other forms of misbehavior that could have serious implications.
What Are Adversarial Attacks?
Adversarial attacks are deliberate attempts by individuals or algorithms to confuse or deceive AI systems. In the context of large language models, these attacks involve inputting data that is specifically crafted to trigger the model into producing incorrect or biased responses. The goal of these attacks can range from benign mischief to more malicious intent, such as spreading false information or manipulating public opinion.
How Adversarial Algorithms Probe Language Models
Adversarial algorithms are sophisticated programs that systematically test and exploit the vulnerabilities of AI models. By probing models like GPT-4 with a series of inputs and analyzing the outputs, these algorithms can identify patterns or weaknesses that can be used to induce the model to make errors. This could involve using unexpected combinations of words, injecting subtle prompts, or exploiting biases within the training data of the model.
The Impact of Adversarial Attacks on AI
The impacts of adversarial attacks on AI, especially in the realm of large language models, are far-reaching. Such attacks can undermine trust in AI applications, distort the truth in digital communications, and potentially cause real-world harm if the information is used to make important decisions. Therefore, safeguarding these models against adversarial attacks is not just a technical challenge but also an ethical imperative.
Strategies for Defending Against Adversarial Attacks
Developing robust defenses against adversarial attacks is a critical area of research in AI. Here are some strategies currently being explored:
- Adversarial Training: This involves including adversarial examples in the training process, helping the model to recognize and resist such attacks.
- Input Sanitization: Implementing checks to identify and modify inputs that are likely to be adversarial before they’re processed by the model.
- Model Regularization: Adjusting the model to be less sensitive to small perturbations in the input that could trigger misbehavior.
- Monitoring and Response Systems: Continuously monitoring model outputs for signs of adversarial attacks and having protocols in place to respond quickly.
Tools and Resources for Understanding Adversarial AI
For those interested in delving deeper into the subject of adversarial AI, there are several resources and tools available. Books such as “Adversarial Machine Learning” can provide a solid foundation in understanding the complexities of these attacks and defenses. You can find this and other related books on Amazon.
Additionally, online courses and tutorials can offer practical experience in dealing with adversarial algorithms. Platforms like Coursera or edX often have specialized courses in cybersecurity and AI that cover these topics.
Conclusion
As AI continues to advance, the arms race between adversarial attacks and defenses will likely intensify. Large language models like OpenAI’s GPT-4 are powerful tools, but they are not impervious to manipulation. Understanding the nature of adversarial attacks and investing in robust defense mechanisms is essential for ensuring the responsible and safe deployment of AI technologies. By staying informed and prepared, developers, businesses, and users can mitigate the risks posed by adversarial algorithms and harness the full potential of AI with confidence.
For those looking to protect their AI systems or simply learn more about adversarial machine learning, consider exploring the resources mentioned above and stay updated on the latest research and developments in the field.