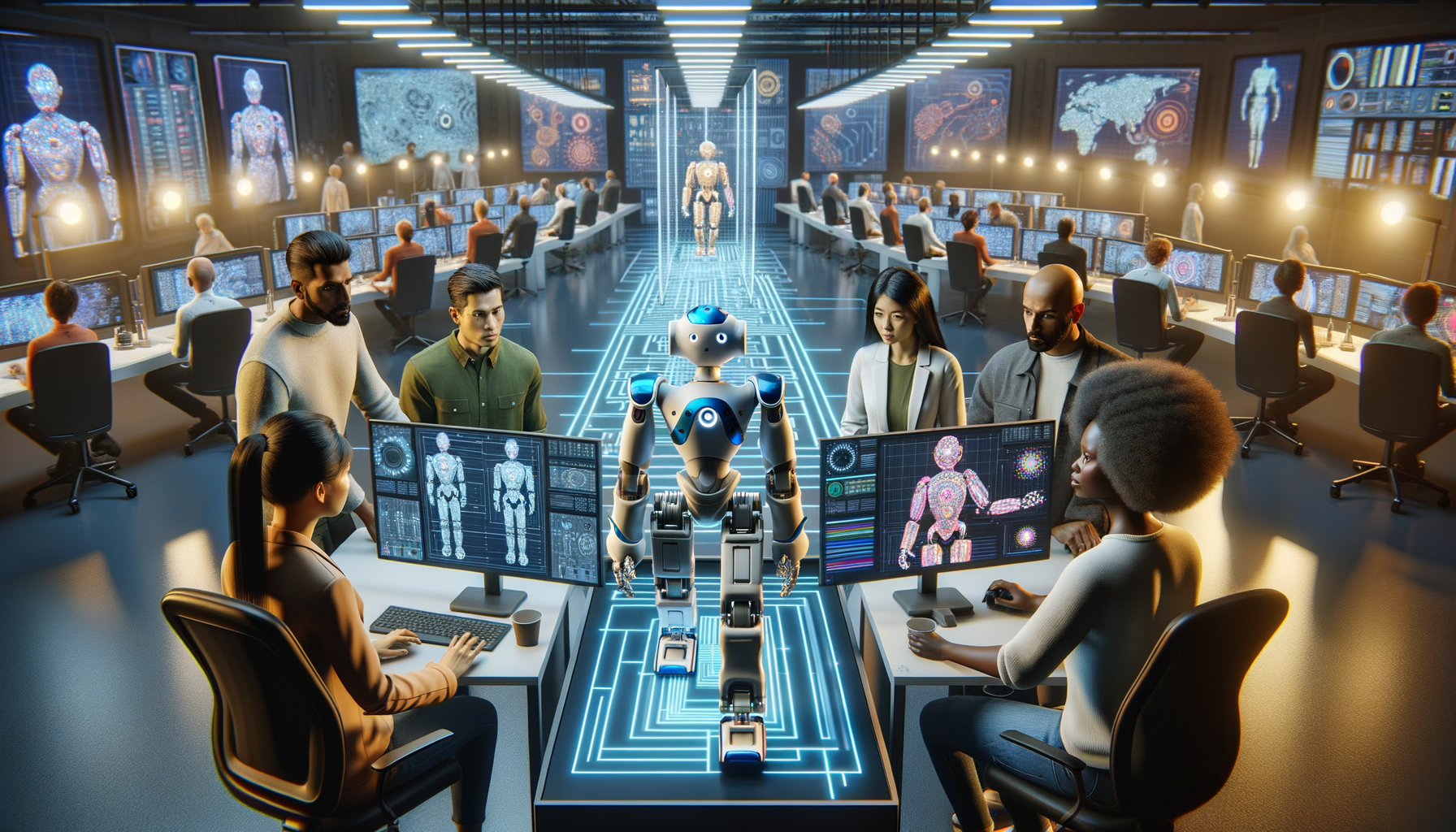
Revolutionizing AI Training: Crowdsourced Data and Reinforcement Learning
In the rapidly evolving world of artificial intelligence (AI), a groundbreaking technique is making waves by leveraging the power of collective human intelligence to train AI agents more efficiently. This method combines the strengths of reinforcement learning (RL) with insights gathered from non-expert humans, crowdsourced asynchronously. The result is an AI that not only learns faster but also performs tasks with a higher degree of proficiency than those trained with traditional methods.
Understanding Reinforcement Learning and Crowdsourced Data
Before diving into the intricacies of this new approach, it’s important to understand the basics of reinforcement learning and the role of human-sourced data in AI training.
Reinforcement Learning: At its core, RL is a type of machine learning where an AI agent learns to make decisions by performing actions in an environment to achieve a goal. The agent receives feedback in the form of rewards or penalties, which guides its learning process. Over time, the agent learns to maximize rewards and thus improve its performance on the given task.
Crowdsourced Data: Crowdsourcing involves gathering information or input from a large group of people, typically from the online community, rather than relying on a small, expert group. When applied to AI training, it means that non-expert humans contribute data or insights that can be used to guide the learning process of an AI agent.
The New Technique: Asynchronous Crowdsourcing in AI Training
The novel technique in question allows AI agents to be guided by data that is crowdsourced asynchronously from a vast pool of non-expert human users. This data is then incorporated into the reinforcement learning process. The asynchronous aspect means that human input can be gathered at different times and does not require real-time interaction with the AI, making the process more flexible and scalable.
Benefits of Asynchronous Crowdsourced Data in RL
- Increased Diversity of Data: By tapping into the knowledge and experiences of a large, diverse group of people, the AI can learn from a wider range of scenarios and responses, leading to more robust learning outcomes.
- Enhanced Learning Speed: The influx of human-provided data can help the AI agent overcome learning bottlenecks more quickly, as it can draw upon the collective problem-solving abilities of the crowd.
- Improved Performance: With a richer dataset that includes human intuition and strategies, AI agents can achieve a higher level of task proficiency, often surpassing what can be achieved through RL alone.
Applications and Implications
The potential applications for this technique are vast, ranging from autonomous vehicles that learn to navigate complex environments to customer service bots that can better understand and respond to human needs. In any domain where human-like decision-making and adaptability are desired, this method could significantly enhance AI performance.
Moreover, this approach has profound implications for the field of AI research. It suggests that the collective intelligence of non-experts can be a powerful resource for training AI, democratizing the process and potentially leading to more ethical and representative AI systems.
Getting Started with AI and Machine Learning
For those interested in exploring the world of AI and machine learning, there are several resources available. Books like “Artificial Intelligence: A Modern Approach” offer comprehensive insights into the field, while online courses can provide hands-on experience with RL and other machine learning methods.
If you’re looking to dive into the technical aspects of AI, consider purchasing some of the top books on the subject. You can find a wide selection by searching Artificial Intelligence Books on Amazon.
Conclusion
The integration of asynchronously crowdsourced data into reinforcement learning signifies a bold step forward in AI training. By harnessing the collective insights of humans from around the world, we can create AI agents that learn faster, perform better, and perhaps even exhibit a touch of human wisdom. As this technique continues to develop, it may well redefine the boundaries of what’s possible in the realm of artificial intelligence.
Stay tuned to the latest in AI research and techniques by following expert blogs and participating in online forums. The future of AI is collaborative, and your contributions could help shape the intelligence of tomorrow.