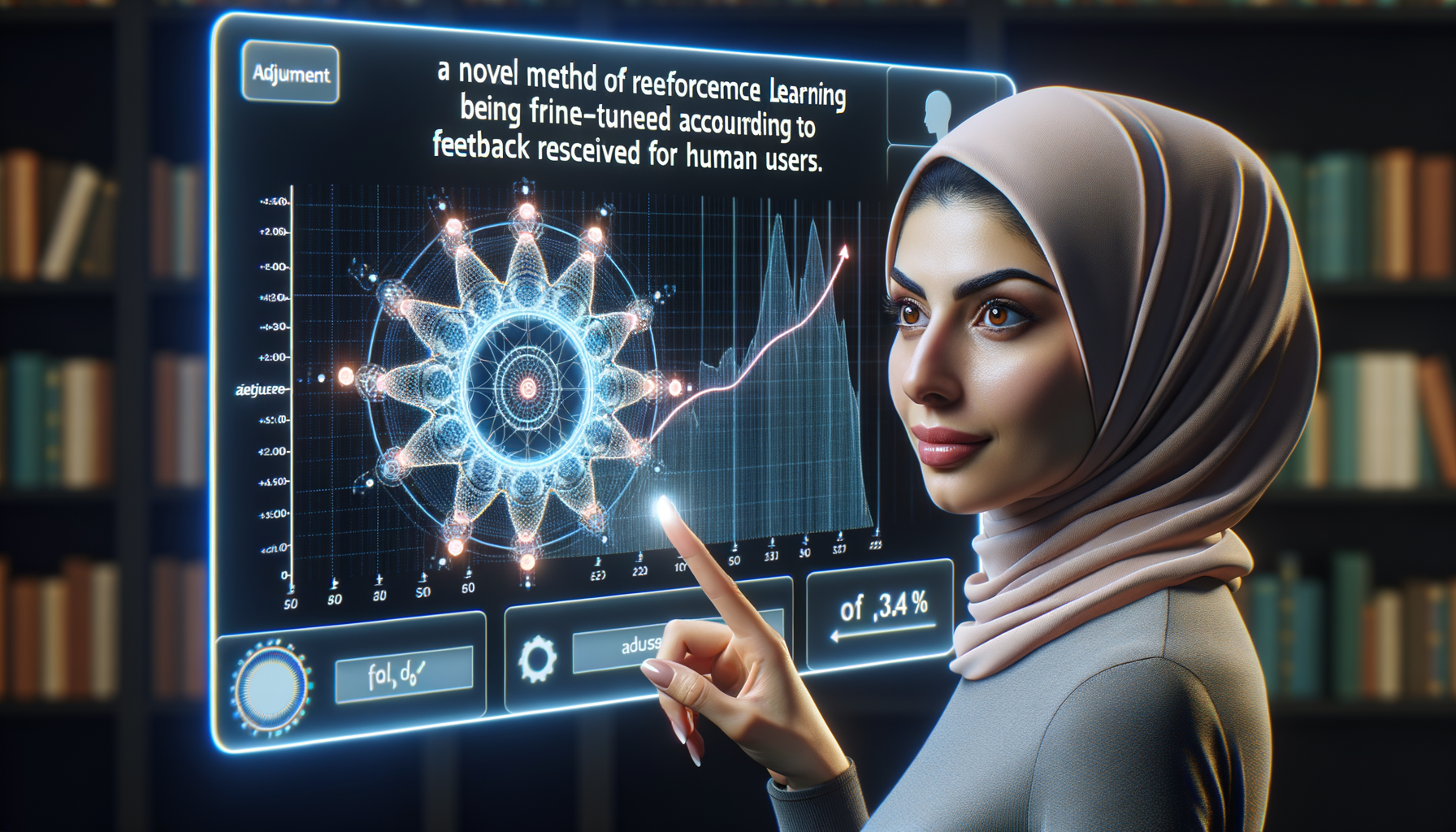
Revolutionizing Error Correction in AI with RLIF: A Simpler Path to Perfection
In the ever-evolving landscape of artificial intelligence, researchers are constantly seeking innovative ways to enhance machine learning models. One such groundbreaking development is the Reinforcement Learning Informed by Failure (RLIF) method. This approach is based on an intuitive yet powerful idea: it is often more straightforward to identify mistakes than to perform error-free actions. In this blog post, we’ll delve into the intricacies of RLIF, explore its potential applications, and examine how it could redefine the future of AI.
Understanding RLIF: The Philosophy Behind Learning from Mistakes
RLIF operates on the principle that learning from failures can be a more efficient path to achieving expertise. Traditional reinforcement learning models focus on rewarding successful outcomes, which can be a slow and complex process, especially when the correct actions are not easily discernible. RLIF, on the other hand, emphasizes the detection and understanding of errors, using these insights to guide the learning process.
By identifying what not to do, AI models can narrow down the range of possible correct actions, effectively speeding up the learning curve. This paradigm shift not only simplifies the training process but also has the potential to create more robust AI systems that can better generalize from their experiences.
Applications of RLIF in Various Industries
The RLIF method can be applied to a multitude of sectors where error recognition plays a crucial role.
Healthcare:
In medical diagnostics, RLIF can assist in developing AI that can quickly identify diagnostic errors, leading to more accurate patient assessments and treatments.
Manufacturing:
Quality control can benefit from RLIF by creating AI systems that are adept at spotting defects and production errors, ensuring higher product standards and reducing waste.
Finance:
Fraud detection algorithms can be enhanced with RLIF, allowing for more precise identification of fraudulent transactions by learning from previous mistakes.
Customer Service:
Chatbots and virtual assistants can use RLIF to better recognize and correct misunderstandings or errors in communication, leading to improved customer interactions.
Implementing RLIF in Your AI Projects
For those interested in incorporating RLIF into their AI projects, there are several resources and tools available that can be instrumental in this pursuit. While there isn’t a one-size-fits-all product for RLIF, AI developers can utilize various machine learning platforms and frameworks that support reinforcement learning to design their own RLIF-based models.
For beginners, books on reinforcement learning can offer a solid foundation in the principles that underpin RLIF. One such resource is “Reinforcement Learning: An Introduction” by Richard S. Sutton and Andrew G. Barto, which provides a comprehensive overview of the field. You can find this book on Amazon by following this link: Reinforcement Learning: An Introduction.
Challenges and Future Directions
While RLIF presents a promising approach, it is not without challenges. One of the main obstacles is ensuring that AI systems can accurately recognize errors in a diverse range of scenarios. Additionally, balancing the focus between error recognition and successful action execution remains a critical aspect of model training.
Looking ahead, research into RLIF will likely delve deeper into how AI can learn from complex and nuanced errors, further refining the error recognition capabilities of machine learning models. As the method matures, we can expect to see more sophisticated AI systems that excel at learning from their mistakes, paving the way for a new era of efficient and effective machine learning.
Conclusion
RLIF symbolizes a significant shift in the approach to training AI systems. By leveraging the simplicity of error recognition, RLIF has the potential to streamline the learning process and produce more capable and adaptable AI models. As the field continues to explore this method, we may witness a transformation in how AI learns and evolves, reinforcing the idea that sometimes, the path to perfection is paved with mistakes.
Stay tuned to this space for more updates on RLIF and other cutting-edge AI research developments. If you’re an AI enthusiast or a professional looking to expand your knowledge, consider exploring the resources mentioned and joining the conversation on how RLIF is reshaping the AI landscape.